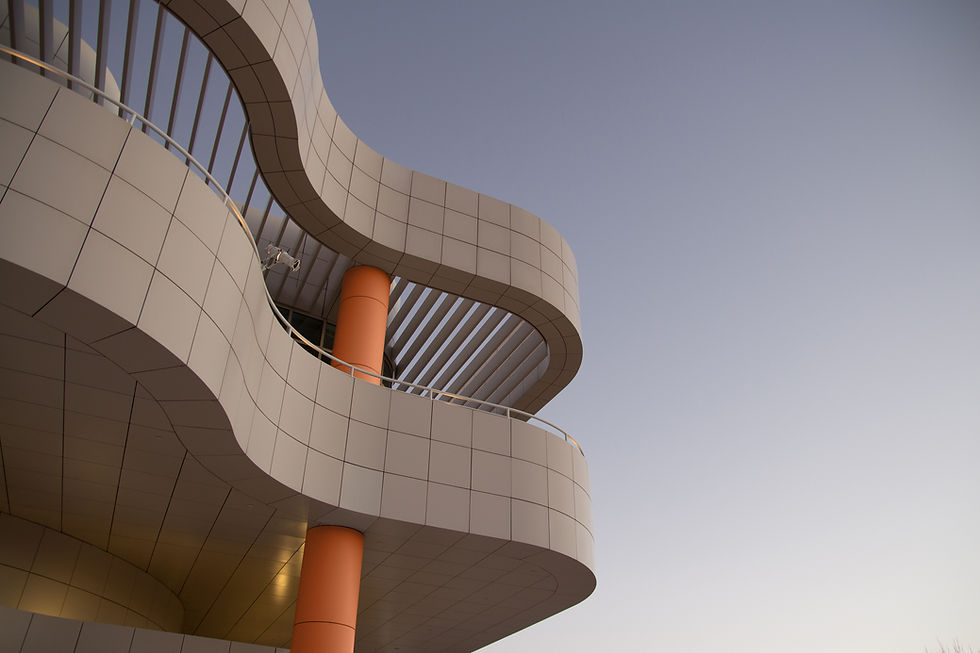
Data Annotation: Unlocking Machine Learning Potential
0
18
0
Imagine standing at the forefront of technological innovation.
You are tasked with creating a sophisticated AI, pivotal to your company's future.
The cornerstone of success relies on precise data annotation—transforming raw data into meaningful inputs that power advanced machine learning models with unparalleled efficacy.
Data annotation is the key.
Understanding Data Annotation
Data annotation lies at the heart of AI.
Imagine a painter choosing colors, tones, and shades. Data annotation is similar, allowing machines to perceive and understand structured input. Through annotating data, we define and label elements, enabling AI to recognize patterns and make informed predictions. Labeling, thus, is not just a technical task but a foundational element of crafting intelligent systems.
High-quality annotation ensures robust model training.
Machine learning performance hinges on - not just the available data - but well-annotated and context-rich labeled datasets.
It's about transforming abstract information into meaningful interconnected experiences, equipping AI to achieve greater precision. As we advance towards 2023 and beyond, the demand for refined annotation increases. Seek innovative solutions, promoting engagement that repeatedly reshapes your data annotation strategy for optimal machine learning progression.
Importance in Machine Learning
Data annotation is a pivotal component in the success of machine learning models. It provides the groundwork for these models to interpret and understand the complexities of data, ensuring accuracy and efficiency.
In 2016, AlphaGo's success, a machine learning algorithm developed by DeepMind, showcased how annotated data could help AI systems navigate complex decision trees—a feat once thought impossible. The system thrived on the meticulously labeled data, which enabled it to learn the game with formidable precision.
Today, it's not enough for models to learn; they must excel in interpreting the subtleties present within diverse datasets. Data annotation remains the cornerstone of this evolution, allowing artificial intelligence to analyze, predict, and produce outcomes with unparalleled accuracy and relevance. Businesses that harness well-annotated data are poised to unlock the full potential of machine learning.
Leaders across various sectors are now acutely aware that their future success depends on the wealth and quality of their annotated data. The precision driven by annotation is not simply an asset but a necessity in an increasingly data-driven world, offering a competitive edge and opening new avenues for innovation.
As we look ahead with optimism, data annotation will continue to empower machine learning. Its critical role in providing clear, actionable insights cannot be underestimated.
Types of Data Annotation
Data annotation is a multifaceted process.
In its diverse forms, data annotation transforms raw data into something golden. The myriad techniques employed depend on the specific requirements of machine learning, which can range from computer vision tasks to natural language processing. Therefore, selecting the appropriate type of annotation is paramount in ensuring the efficacy and efficiency of machine learning models.
Text annotation enhances machine comprehension significantly.
By employing methods such as entity recognition, sentiment annotation, or intent detection, text data is transformed into structured information, making it invaluable for applications like chatbots or search engines. This structure imbues the data with a sense of organization, which the machine can navigate more intuitively.
Similarly, image annotation involves tagging images to build machine learning models with enhanced acuity, benefiting autonomous vehicles, healthcare diagnostics, and more. Visual data is crafted into actionable insights, sculpted by processes like bounding box, polygonal segmentation, or 3D point cloud annotation. As tools evolve and our understanding deepens, the efficacy of image annotation in revolutionizing industries continues to unfold, fortifying the path toward an innovative future.
Image Annotation Techniques
Diverse image annotation techniques, a catalyst for groundbreaking innovations, unlock vast potential. Techniques like bounding box enable machines to accurately identify objects, while semantic segmentation provides a detailed pixel-level understanding of images. As we advance, innovative annotation methods further empower machine learning models to navigate complex environments with precision.
Object Detection
Object detection stands as a cornerstone in image annotation, enabling machines to discern and interact with their surroundings adeptly.
By 2025, the object detection market is projected to reach $4.4 billion, underscoring its significance and rapid growth.
Employing sophisticated techniques like bounding boxes and advanced neural networks, object detection empowers computer vision systems in applications from autonomous driving to real-time surveillance, enhancing both efficacy and safety.
Through the precise delineation and labeling of objects within images, data annotation transforms raw visual information into structured datasets, fueling the evolution of machine learning models and pushing the boundaries of what's conceivable today.
Semantic Segmentation
Semantic segmentation revolutionizes image understanding by classifying each pixel into meaningful categories, offering a comprehensive view of the visual scene.
Image Processing: Analyzes every pixel, enhancing context and detail distinction.
Boundary Refinement: Defines precise edges, crucial for accurate scene interpretation.
Category Assignment: Allocates pixels to specific categories, improving recognition tasks.
Data Consistency: Ensures uniformity across datasets, crucial for model training.
This technique facilitates the development of models that excel in tasks requiring minute detail and rich contextual awareness.
By providing pixel-level insights, semantic segmentation fosters sophisticated applications spanning from autonomous navigation to medical imaging.
Text Annotation Methods
Text annotation methods, a linchpin of natural language processing, bring order to chaos. Annotators label vast sets of unstructured text, imbuing them with a nuanced layer of understanding that machines can decipher, paving the way for smarter, more intuitive learning systems.
Named Entity Recognition
Named Entity Recognition (NER) identifies key information from text, enhancing machine comprehension and allowing systems to intelligently process substantial data volumes. Crucial for transforming raw text into meaningful insights.
It efficiently extracts names, organizations, locations from diverse textual data.
NER stands as a transformative tool in developing intelligent applications capable of providing a cursory analysis of numerous documents that contain various entities like products, companies, and people, ultimately facilitating robust search algorithms, personalized recommendations, and content categorization.
The efficacy of NER plays a vital role in a myriad of industries such as finance, healthcare, and technology by streamlining information retrieval and decision-making processes. As organizations increasingly depend on automated systems for handling extensive volumes of text data, NER's precision and adaptability ensure it stands as "central" to achieving operational excellence and insightful innovation.
Sentiment Analysis
Sentiment analysis is the gateway to understanding human emotions through machine learning, enabling systems to identify and process sentiment-laden data.
Data Collection: Gather data from various sources such as social media, reviews, and news articles.
Data Annotation: Label data with appropriate sentiment categories, such as positive, negative, or neutral.
Model Training: Use annotated data to train sentiment analysis algorithms.
Model Evaluation: Assess the accuracy of the model by comparing predicted sentiment against human-evaluated sentiment.
Deployment and Feedback: Implement the model in real-world applications and refine it based on user feedback.
By deciphering emotions within text, sentiment analysis unlocks valuable insights beneficial for numerous applications.
This advanced capability is pivotal in enhancing user experience, enabling personalized interactions, and informing strategic decisions across industries.
Audio Annotation Strategies
Audio annotation is the cornerstone of transforming audio content, a medium of unstructured information, into data-rich resources. Annotating audio not only aids in extracting valuable insights but also enhances the precision of machine learning models reliant on sound, speech, or acoustic data.
In the realm of audio, the annotation process can involve tasks like "speaker labeling". This enables systems to identify who is speaking at a given moment, thereby adding layers of context to the audio data. By meticulously annotating audio to distinguish between various speakers, the foundation is laid for more advanced audio recognition capabilities essential in applications ranging from virtual assistants to security systems.
Speech Recognition
In the modern world, speech recognition systems have become pivotal in bridging the gap between human interaction and technology.
For these systems to perform at their best, data annotation plays a crucial role. The process involves transcribing audio inputs into text, ensuring that the systems accurately understand and respond to spoken language. Through diligent and comprehensive data annotation, these systems can tackle the complexities of accents, dialects, and colloquial expressions with higher proficiency and reduced error rates.
Speech recognition technology thrives on the depth of annotated datasets it is trained on. Annotators focus on various linguistic elements such as context, tone, and intent, which enriches the learning capabilities of AI systems. This meticulous approach allows speech recognition models to evolve and adapt to an ever-diverse linguistic landscape.
As technology advances, the potential for speech recognition continues to grow exponentially, unlocking seamless, intuitive interactions between people and machines. From streamlining customer service to providing accessibility solutions for individuals with disabilities, the implications are transformative. By investing in robust data annotation processes, we empower these systems to achieve new heights of accuracy and efficiency, continually pushing the boundaries of what is possible in machine-human interaction.
Acoustic Scene Analysis
Listen closely; the world is speaking.
Acoustic scene analysis is an exciting frontier in data-driven sound interpretation. This field involves using machine learning models to understand the vast tapestry of sounds present in our environment, allowing us to categorize and make sense of diverse audio inputs. Through data annotation, specific sounds are tagged and labeled to train algorithms to accurately identify and respond to the acoustic characteristics of different scenes.
Crucial for intelligent systems, this technology perceives sounds beyond words.
Applications range from enhancing security systems to facilitating hearing aids - by distinguishing specific sounds, such as a doorbell from background noise - to help users better interact with their environment. Highly sophisticated algorithms trained with annotated audio data are pushing the capabilities of automatic sound recognition further.
With continuous advancements in computational prowess, annotated sound datasets boast remarkable potential. Technologies once seen as futuristic are now commonplace thanks to cutting-edge research and development. Acoustic scene analysis, when empowered by comprehensive data annotation, is revolutionizing how we perceive and interact with our auditory world.
Video Annotation Approaches
Video annotation, a crucial component of machine learning, frames the way for robust algorithm development. By assigning labels to various video elements, we facilitate the training of models that can intelligently interpret and respond to the dynamic visual cues within videos.
In exploring the intricacies of a video frame, techniques such as "semantic segmentation" come into play. This involves dividing a video into smaller segments, allowing models to identify individual objects. To this end, the automation of this process, through innovations in AI, promises to elevate the efficacy of video analysis, unlocking new dimensions in visual comprehension.
Frame-by-Frame Labeling
Frame-by-frame labeling offers a meticulous approach to annotating individual video frames with precision, thus greatly enhancing the nuanced understanding of the content.
This method involves assigning specific labels to each frame within a sequence.
This precision enables models to capture subtle variations across frames, establishing a detailed narrative of events with a level of granularity that is unparalleled, thereby improving accuracy, efficiency, and adaptability in various applications.
Employing frame-by-frame labeling is undoubtedly a cornerstone in the evolution of video data annotation. It is indispensable in domains requiring exceptional detail, such as medical imaging and autonomous driving systems, thus becoming a linchpin solution for advancing machine learning technologies. As models evolve, precision now is "key" to embracing this innovative solution for future growth.
Tools for Data Annotation
Effective data annotation, when harnessed through the right tools, transforms raw datasets into rich resources that empower machine learning models.
These tools, as varied as the datasets themselves, offer a structured approach tailored for maximum efficiency, thereby enabling annotation teams to focus on creating accurate and meaningful datasets. Platforms such as Labelbox and RectLabel provide specialized interfaces that cater to different data types, allowing for seamless integration and precise annotation. They streamline the annotating process with features that accommodate collaboration, thus multiplying the effectiveness of data teams worldwide.
Moreover, choosing the ideal tool is a pivotal aspect of any annotation strategy. In making this selection, factors such as the user interface, compatibility with dataset formats, and scale of automation need to be considered as they significantly influence workflow and data quality.
Ultimately, the right annotation tools not only support the technical demands of machine learning but also inspire a new echelon of innovation. They empower teams to utilize their creativity and expertise, resulting in data that drives technological breakthroughs and enriches AI systems. As we seek to redefine machine capabilities, investing in these tools becomes a strategic pillar supporting our collective aspiration for transformative artificial intelligence.
Challenges in Data Annotation
Data annotation, despite its monumental benefits, comes with inherent challenges that require innovation and strategic approaches to surmount.
Firstly, one of the predominant hurdles is the sheer volume of data that needs annotation, which can be overwhelming. Ensuring high-quality, accurate annotations demands significant attention to detail, stringent quality control measures, and robust validation processes. These tasks can be time-consuming and resource-intensive, requiring astute management and allocation of both human and computational resources to ensure sustainability in the long term.
Moreover, achieving consistency across annotations remains a persistent challenge. As different annotators may interpret data in varying ways, establishing uniform guidelines and training protocols becomes essential. By promoting a uniform understanding of annotation goals and methodologies, organizations can enhance the accuracy and reliability of their annotated datasets.
Finally, the ongoing evolution of machine learning models necessitates continuous updates and revisions to annotated data. This dynamic landscape requires adaptable annotation frameworks capable of evolving with emerging technologies and methodologies. Investing in flexible and scalable annotation systems is crucial to maintaining a competitive edge and fostering advancements in AI while also navigating the complexities of data annotation with resilience and foresight.
Best Practices for Accurate Annotation
Accuracy in data annotation forms the bedrock of effective machine learning and AI applications. By adopting best practices, we can ensure consistency, efficiency, and excellence in results.
Prioritize a systematic approach underpinned by rigorous training sessions. These are designed to align annotators' comprehension.
Thorough training modules cultivate a deep-seated understanding among annotators, ensuring they grasp the nuances of data, thereby promoting uniformity and minimizing discrepancies. This fosters a shared vision that aligns annotations with desired outcomes.
Furthermore, continuous feedback loops and error analysis serve as vital components in refining annotation accuracy. By embracing an iterative process that allows ongoing evaluation and refinement, organizations can swiftly adapt and mitigate ambiguities, ensuring that the annotated data remains as precise and effective as possible. This proactive strategy not only sharpens AI models but also instills a dynamic culture of innovation and improvement.
Impact on Model Performance
The significance of data annotation cannot be overstated as it directly influences machine learning model efficacy. Properly annotated data serves as the crucial foundation that determines the predictive power and accuracy of a model.
When annotation quality is high, models can more accurately learn patterns and relationships, leading to enhanced outcomes.
High-quality data annotations are akin to clear lenses for algorithms, magnifying their capacity to comprehend and predict. Accurate annotations translate to fewer errors.
This, in turn, results in more reliable outputs and insights, thereby increasing model precision and trustworthiness. Consistent, well-defined annotations mean fewer re-training sessions.
Such improvements bolster model performance, providing organizations with a substantial competitive edge as insights become more actionable and precise. Consequently, businesses can leverage this performance to harness new opportunities and drive growth.
Future Trends in Data Annotation
As we embark on the horizon of next-generation machine learning innovations, the realm of data annotation is set to witness remarkable evolution, one that will redefine the landscape of AI technologies.
Automation and human-in-the-loop strategies will exponentially enhance data quality.
This synergy between cutting-edge automation and adept human oversight promises a future where annotation processes are not just faster but also imbued with unprecedented accuracy, consistency, and context, empowering organizations to unlock new dimensions of insight.
The seamless adoption of AI-driven assistive tools for annotators will become a cornerstone in this transformative landscape, offering efficiency and precision in annotating vast datasets. These innovations will not only accelerate the annotation pipeline but also ensure superior quality through adaptive learning and real-time feedback systems. Today, data annotation is the backbone driving tomorrow's machine intelligence breakthroughs.
Frequently Asked Questions (FAQ)
What is data annotation?
Data annotation is the process of labeling data to make it understandable for machine learning models. It involves adding metadata to various data types, such as text, images, audio, and video, to help algorithms recognize patterns and make informed decisions.
Why is data annotation important?
Data annotation is crucial because it provides the foundational training data that machine learning models require to learn and improve. Without accurately annotated data, models would struggle to understand and interpret real-world information, leading to suboptimal performance.
What are the different types of data annotation?
Data annotation encompasses several types, including:
Text Annotation: Involves labeling text data for tasks like sentiment analysis, named entity recognition, and language translation.
Image Annotation: Includes labeling images for object detection, image segmentation, and facial recognition.
Audio Annotation: Entails transcribing and labeling audio data for speech recognition and language processing.
Video Annotation: Involves tagging video frames for object tracking, action recognition, and scene understanding.
How do you ensure the quality of annotated data?
Ensuring high-quality annotated data involves several strategies:
Clear Guidelines: Providing annotators with detailed instructions and examples.
Training: Offering comprehensive training sessions for annotators to understand the task requirements.
Quality Checks: Implementing regular quality control measures, such as peer reviews and automated checks.
Feedback Loops: Establishing a system for continuous feedback and improvement.
What tools are commonly used for data annotation?
There are numerous tools available for data annotation, each catering to different needs. Some popular ones include:
Labelbox: A versatile platform for image, text, and video annotation.
Amazon SageMaker Ground Truth: Offers scalable annotation services with built-in quality control.
SuperAnnotate: Provides collaborative annotation tools for image and video data.
Prodigy: A scriptable annotation tool for text data, ideal for NLP tasks.
How does data annotation impact machine learning models?
Data annotation directly impacts the accuracy and efficiency of machine learning models. Well-annotated data enables models to learn effectively, leading to better predictions and insights. Conversely, poor annotation can result in biased or inaccurate models, undermining their reliability and usefulness.
Can data annotation be automated?
Yes, data annotation can be partially automated using AI-assisted tools. These tools leverage pre-trained models to suggest annotations, which human annotators can then verify and refine. While automation can enhance efficiency, human oversight remains essential to ensure accuracy and context understanding.
What are the challenges in data annotation?
Data annotation presents several challenges, including:
Scalability: Managing large volumes of data efficiently.
Consistency: Maintaining uniformity across annotations.
Complexity: Handling intricate data types and nuanced tasks.
Bias: Avoiding subjective interpretations that could skew model outcomes.
How can I get started with data annotation?
To embark on data annotation, begin by:
Defining Objectives: Clearly outline the goals and requirements of your annotation project.
Choosing Tools: Select appropriate annotation tools that align with your data type and project scope.
Training Annotators: Provide comprehensive training to ensure annotators understand the task.
Implementing Quality Control: Establish robust quality assurance processes to maintain high standards.
By following these steps, you can set a strong foundation for successful data annotation, paving the way for impactful machine learning applications.